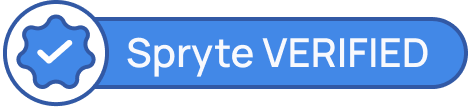
Description
While most companies in the business of drone software for data analytics belong to the energy and construction sector, our client is an ag-tech solution provider with a use case of crop-health monitoring system. We were on the job for refinement and churning out its training data for an AI model that analyses diseases in crops. The company uses drone imagery to fuel their machine learning to come up with insights and hidden patterns that farm managers and agronomists can use to tackle crop stress, diagnose diseases and plan treatments.
Challenge
Drone imaging is capable of producing accurate image location to the millimetre. For our client it means that once the planting is done by farmers, areas with stand gaps can be spotted and replanted as needed, and disease or pest problems can be detected and treated with analysis right away. Also, It is hard to invest time in data labelling with diverse planting patterns with increased crop diversity, less well known produce, and grains which look similar throughout their growth stages. Banking on newer technologies is met with resistance since it could have a case-based or a domino effect on a particular region. Thus, an accurate and exhaustive set of training data that is perfectly annotated was a prerequisite for our client’s AI model. Our client’s farmer market had unique requirements specific to each user’s case since every farmer has diverse planting variations on the field and goes with tailored crop protection measures that suit their fields the best. Thereby, making it a necessity for our client to alter their model as per the needs of its audience; which snowballed into specific labelling instructions for respective imagery datasets.
Solution
For us it meant that the image annotation task at hand was an exhaustive one with precise agronomy knowledge. We provided a dedicated project manager to the client as a single point of contact, who could be given a download of the domain knowledge, business rules of the use case and could be reached out from time to time for checks on work progress. Two small teams of 6 labellers each were trained on the job in the first 3 weeks. Initially our client’s team monitored closely to guide with edge cases and nuanced annotations, but after the 4th week our teams were able to accurately identify and tag differentiated images on a production scale. We also made sure that our choice of annotation platform is judiciously discussed to ensure dataset accuracy, AI enabled pre labelling features and a speedy labelling process.